The Australian Plague Locust Commission (APLC) collaborated with the Centre of Excellence for Biosecurity Risk Analysis (CEBRA) to develop advanced forecasting tools based on machine learning in response to the challenges posed by the Australian plague locust (Chortoicetes terminifera - hereafter referred to as ‘Ct'). Representing a significant advancement in locust management capabilities, the project aims to minimise agricultural losses by allowing the prediction of future locust outbreaks.
Leveraging 37 years of APLC and Member State data, the project utilised Random Forest Classification models to forecast locust nymph presence, developmental stages, and densities. The machine learning process incorporated training on over 300, 000 harmonised locust records to achieve high predictive power for the presence of locust nymphs, which will provide a solid basis for ongoing strategic and operational planning.
The modelling incorporated essential environmental variables, including body temperature (as a function of ambient temperature), precipitation, soil type, woody vegetation cover, and vegetation growth indices, all influencing locust dynamics.
Temperature and rainfall emerged as the most influential variables from the analyses. Identifying the most critical environmental drivers of locust phenology and density is essential for refining predictive accuracy and understanding the ecological processes that underpin locust population dynamics.
A new Ct Habitat Suitability Map was also developed using National Vegetation Information System (NVIS) vegetation classes and ABARES land-use data. This map replaces the previous one, created over twenty years ago. The new map highlights the areas most conducive to Ct habitation and strengthens APLC’s capacity for prioritised surveillance and targeted intervention.
The forecasts produced by the models will also provide one-to-two-week interval predictions across the locust’s range, enabling timely resource allocation for surveillance and early intervention – which will improve operational efficiency and strategic planning.
The project required substantial data harmonisation and processing effort to facilitate the detailed analysis. Approximately half a million locust records were quality-controlled from 1986 to 2022 to produce a ‘gold standard’ locust dataset. Terabytes of high-resolution weather, soil, and vegetation data were processed at a high computational cost. The locust and environmental data were then meshed to create a single database used for the analyses.
The Random Forest Classification models showed strong predictive accuracy, particularly for the first and fifth instar locust nymph stages. However, challenges remain, especially in addressing false absences in the later instars, particularly the fifth instars. This is due to behavioural shifts and increased mobility in these stages.
Future work will focus on improving the accuracy of these aspects and integrating these tools into a new version of APLC’s Web-mapper - which functions as a real-time data dashboard and decision-support system, consolidating locust density, weather, and vegetation data over the past 12 months for operational use.
This project exemplifies the transformative potential of integrating large-scale, high-quality datasets with state-of-the-art artificial intelligence tools to bolster biosecurity outcomes. With these advanced forecasting tools, APLC is better equipped to mitigate locust outbreaks, ultimately helping safeguard Australia’s agricultural productivity.
Image credit: Dr Gordon Berg, Victoria APLC Commissioner (ret.)
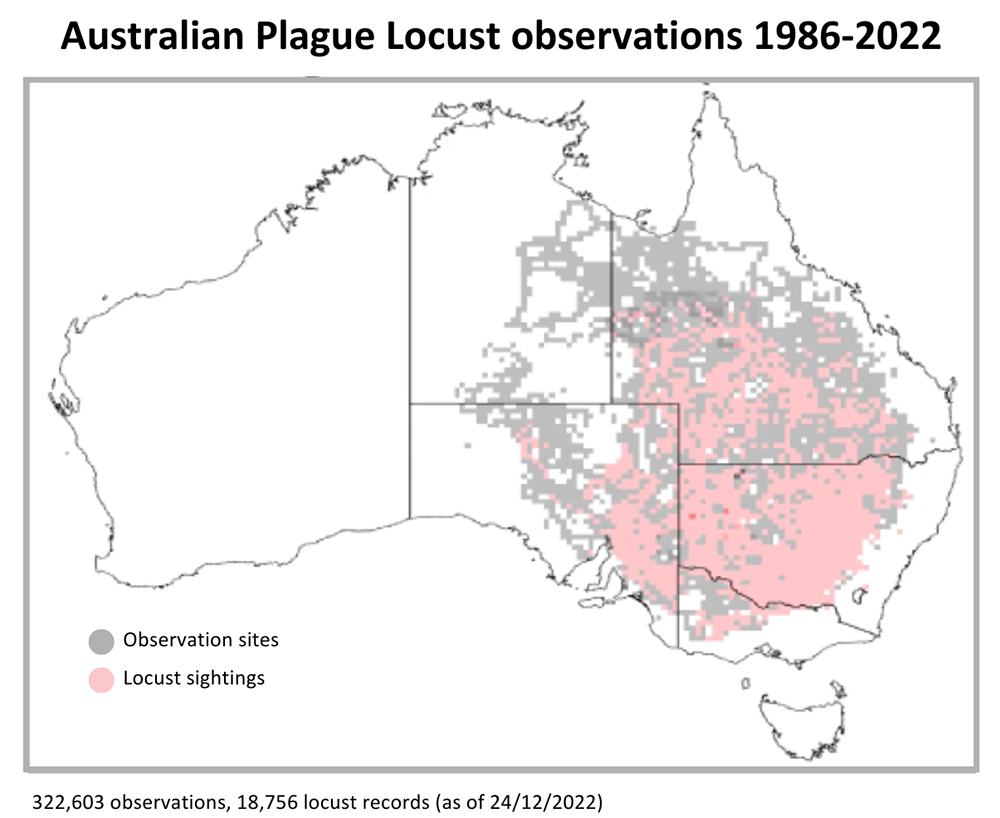
Credit: Baumgartner et al., 2024.
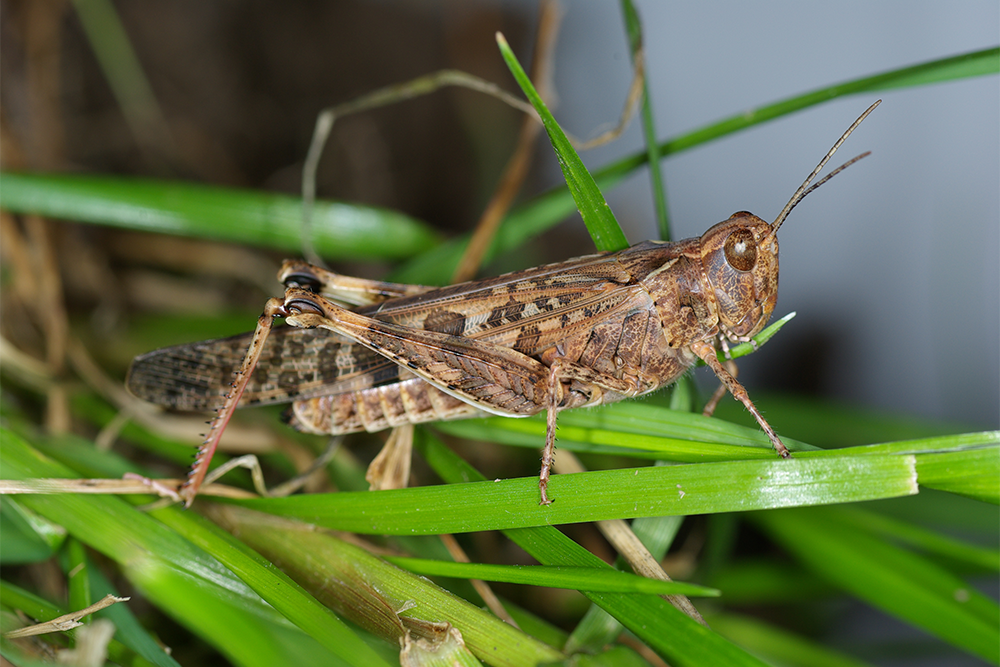
Image credit: Dr Gordon Berg. Victoria APLC Commissioner (ret.)
Reference
Baumgartner, J.B., Spessa, A.C., Deveson, E., and Camac, J.S. (2024). Short-term population forecasting of the Australian Plague Locust (Chortoicetes terminifera). CEBRA project 23A. Technical Report for the Australian Plague Locust Commission, Dept Agriculture, Fisheries and Forestry, Canberra, Australia. 49 pp.